Adetitun D.O.; 1 Oyagbola E.O. 2 Adetomiwa A.A.; 3 & Tomilayo R.B.4
1Department of Microbiology, Faculty of Life Sciences, University of Ilorin, Nigeria.
2Department of Science Laboratory Technology (Microbiology Unit), Kwara State Polytechnic, Ilorin, Nigeria.
*Corresponding Author Email: adetitun.do@unilorin.edu.ng …
Abstract
Rapid industrialization and frequent oil spills have led to severe environmental challenges, particularly petroleum hydrocarbon contamination of soil and water. While microbial degradation is a promising bioremediation strategy, its effectiveness is often unpredictable due to variations in microbial communities and environmental conditions. Artificial intelligence (AI) offers a transformative solution by analyzing vast datasets, identifying patterns, and developing predictive models to optimize bioremediation efforts. By integrating AI with microbial degradation processes, researchers can forecast outcomes, select optimal microbial consortia, and enhance cleanup efficiency. This article explores the role of AI in microbial oil degradation, highlighting recent advancements, challenges, and future research directions. AI-driven approaches can revolutionize bioremediation by making it more efficient, cost-effective, and scalable. Harnessing AI’s predictive capabilities will enable targeted remediation strategies, contributing to a cleaner and healthier environment. Continued interdisciplinary research and innovation are essential to fully leverage AI for sustainable environmental management.
Keywords: Artificial intelligence, microbial degradation, bioremediation, oil contamination, environmental sustainability
1. Introduction
ignificant environmental issues have arisen from rapid industrialisation and frequent oil spills, particularly concerning petroleum hydrocarbon contamination of soil and water (Hemmati et al., 2024). Microbial degradation has emerged as a viable natural approach for minimising the environmental impact of these pollutants. However, forecasting and maximising bioremediation efforts remain challenging due to the complexity and diversity of microbial populations as well as the wide range of environmental conditions.
With its potent predictive capabilities, artificial intelligence (AI) presents a game-changing solution to this problem. By utilising machine learning techniques, AI can develop prediction models from large datasets on microbial behaviour, environmental conditions, and degradation pathways. Such models can forecast the effectiveness of bioremediation strategies in various settings, assist in selecting the optimal microbial consortia, and enhance the overall performance of cleanup operations for oil contamination.
This article examines the integration of AI into the microbial degradation of oil contamination, emphasising its potential to transform environmental bioremediation practices. We review the current state of AI applications in this field, discuss encountered challenges, and outline potential avenues for further research and advancement. The ultimate goal is to contribute to a cleaner and healthier environment by providing a comprehensive overview of how AI can be harnessed to increase the predictability and success of microbial degradation processes.
2. Background
Oil contamination is a major environmental concern, especially in areas with high levels of industrial activity, oil exploration, and transportation (Adetitun, 2020). The persistent presence of petroleum hydrocarbons in soil and water poses significant risks to local economies, human health, and ecosystems (Mohanta et al., 2024). Traditional remediation techniques—such as physical removal and chemical treatments—often prove insufficient because of their high cost, potential for secondary pollution, and partial degradation of toxic compounds (Navina et al., 2024). In this context, microbial degradation has emerged as a promising alternative for bioremediation of oil‐contaminated environments (Tedesco et al., 2024).
Microbial degradation harnesses the natural metabolic activities of specific microorganisms, including bacteria, fungi, and archaea, to convert complex hydrocarbons into less hazardous compounds (Amini et al., 2024). These microbes break down hydrocarbons into simpler molecules such as carbon dioxide, water, and biomass, which they then use as sources of carbon and energy (Gertsen et al., 2024). However, the efficiency of microbial degradation is influenced by several factors, including the type and concentration of hydrocarbons, the composition of the indigenous microbial community, environmental conditions, and nutrient availability (Siddique et al., 2024).
The unpredictable nature of the process makes microbial degradation a challenging bioremediation technique to implement. Bioremediation projects often depend on the precise alignment of multiple variables due to the intricate interactions between microorganisms and hydrocarbons (Barbinta-Patrascu et al., 2024). Consequently, there is a growing interest in employing AI to enhance the efficiency and predictability of microbial degradation (Taiwo et al., 2024).
AI offers a revolutionary approach to bioremediation through its capacity for data processing, pattern recognition, and predictive modelling. By analysing vast datasets—including genomic data, environmental parameters, and historical bioremediation outcomes—AI can identify patterns and relationships that may elude traditional methods. This capability enables the optimisation of the bioremediation process through predictive models that forecast microbial community behaviour under specific conditions (Baskaran & Byun, 2024).
Environmental biotechnology has made great strides with the application of AI in predicting microbial degradation of oil contamination. Researchers and environmental specialists can now more accurately anticipate outcomes, comprehend the intricate dynamics of microbial degradation, and develop more efficient, site-specific bioremediation strategies. This article explores the current applications of AI in microbial degradation, highlights key technological advancements, and discusses how AI may ultimately transform the environmental remediation industry.
2. Artificial Intelligence (AI) Techniques in Microbial Degradation
Artificial intelligence (AI) is currently receiving substantial attention in various fields, including pattern recognition, medical diagnosis, image processing, intelligence search, autonomous programming, and robotics (Fan et al., 2018). AI is an interdisciplinary field that applies innovative approaches to solve problems cost-effectively (Baum et al., 2021; Patowary et al., 2023). Advances in high-quality software development, increased computational speed, and ongoing information technology improvements have led to the creation of fast and accurate supercomputers.
Crude petroleum oil spills seriously endanger the environment and pose hazards to both flora and fauna worldwide. Bioremediation (see Figure 1) is one of the many environmentally benign and economically viable methods employed to mitigate fossil fuel pollution (Navina et al., 2024). However, the hydrophobic and recalcitrant nature of oily components often limits their accessibility to the biological agents responsible for the degradation process. In recent years, the utilisation of nanoparticles for oil pollution restoration has gained popularity due to their unique properties (Singh et al., 2020). Combining nanotechnology with bioremediation gives rise to “nanobioremediation”, a promising technology that is anticipated to overcome the shortcomings of conventional bioremediation. Moreover, the integration of AI with these traditional remediation methods can lead to effective, rapid, reliable, and highly accurate strategies for rehabilitating oil-contaminated systems (Patowary et al., 2023).
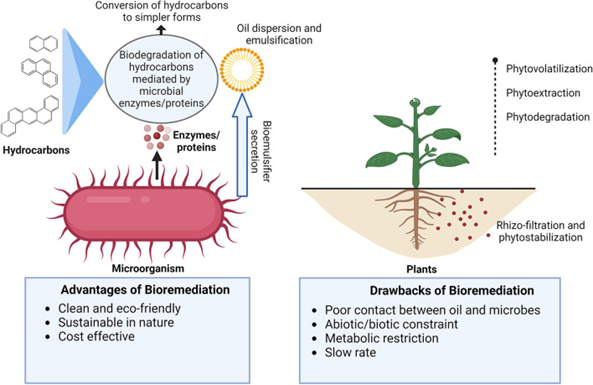
Figure 1: The process of bioremediation of hydrocarbon pollutants from contaminated locations (Patowary et al., 2023).
3.1 Machine Learning (ML), Deep Learning (DL), Data Mining, Predictive Modeling
AI substantially contributes by analysing large volumes of data collected via Internet of Things (IoT) devices, thereby identifying patterns and correlations that may be missed by human analysis (Paramesha et al., 2024). This enhanced understanding leads to improved contamination source tracing and the development of more effective cleanup techniques. AI models can forecast the outcomes of various bioremediation strategies by accounting for factors such as pollutant type, environmental conditions, and microbial activity.
Furthermore, AI can dynamically adjust critical bioremediation parameters—such as microbial inoculation, nutrient supply, and oxygen levels—in response to real-time data (Chung & Dhar, 2024). Future advancements in AI, particularly in sophisticated machine learning methods, are expected to facilitate the development of adaptive bioremediation systems that continuously improve through learning from operational data. The integration of robotics with AI may also yield autonomous systems capable of performing complex tasks with minimal human intervention (Kuppan et al., 2024).
In recent decades, bioremediation of hydrocarbons (see Figure 1) has garnered significant global interest, including applications in Antarctic maritime environments.
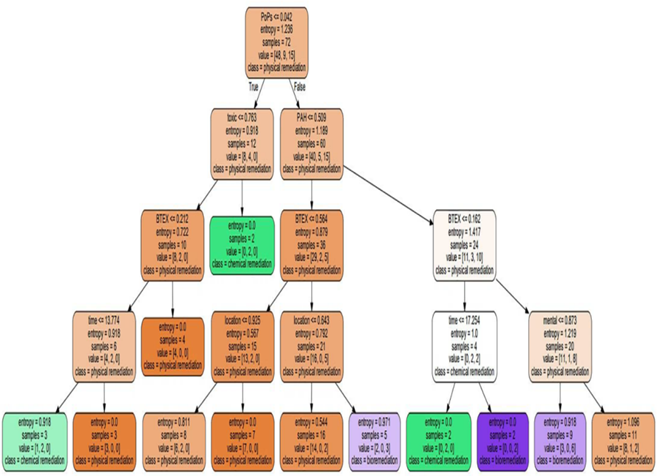
Figure 2: Texas and California decision tree models for addressing oil pollution (Li et al. 2022)
Two prominent areas of AI that have recently received considerable attention are machine learning (ML) and deep learning (DL). Machine learning automates the development of analytical models, enabling computers to learn from data without explicit programming. In contrast, deep learning—a subset of machine learning—employs neural networks to solve complex problems by learning from unstructured and unlabelled data (Sharifani & Amini, 2023). While machine learning utilises statistical approaches for data analysis, deep learning leverages neural networks to learn from extensive datasets (Mahyar & Bozorgasl, 2023; Sharifani & Amini, 2023).
For instance, the Cubist algorithm (Figure 3) combines the principles outlined by Quinlan (1992, 1993) in a prediction-oriented regression model. Cubist initially constructs a decision tree and subsequently collapses each path into a rule. A regression model is then fitted for each rule-defined data subset. This approach is closely related to the M5 (Model Tree) methodology.
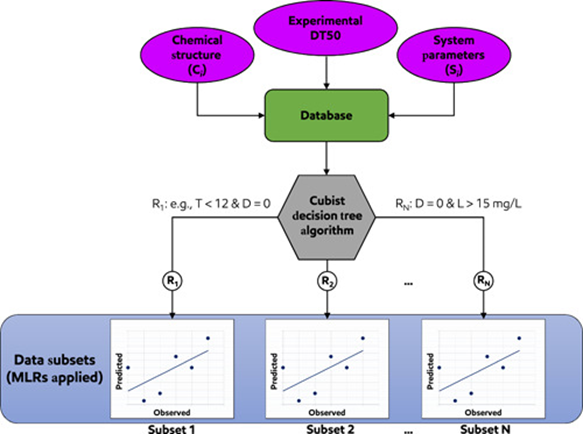
Figure 3: The Cubist decision tree machine-learning methodology for the system-integrated model (HC-BioSIM). Chemical structure (Ci), system (Si) characteristics, and experimental disappearance time (DT50) values (labels used to train the model) are provided as user-defined inputs. White circles represent the model-defined rules (Ri) used to partition the dataset, and the blue box indicates the terminal subset “nodes” where multiple linear regressions (MLRs) are applied to forecast DT50 values. (Adapted from Kuhn et al., 2012).
In‐silico methods have been employed alongside computational frameworks to facilitate predictive bioremediation, with the dual aims of removing contaminants and assessing the toxicity and potential degradation pathways of recalcitrant chemicals (Lyu et al., 2024). Emerging pollutants from a myriad of industries have placed both the environment and public health at significant risk. Current bioremediation techniques frequently fall short or prove unsustainable when tasked with mitigating hazardous contaminants.
Traditional remediation approaches often suffer from a lack of precise and critical information regarding biodegradation. This deficiency in our understanding of biotransformed substances has prompted the search for alternative techniques (Liu et al., 2024). Indeed, there is scant literature addressing the toxicity profiles and altered products of biodegradation processes. In response, a range of computational or in‐silico technologies has evolved, which are now recognised as viable approaches for bioremediation (Rawal et al., 2024).
Key components of predictive biodegradation include Quantitative Structure-Activity Relationship (QSAR) and Quantitative Structure-Biodegradation Relationship (QSBR) model systems. These systems incorporate techniques such as molecular docking, molecular dynamics modelling, and biodegradation pathway prediction. In addition, methods based on machine learning (ML), artificial neural networks (ANN), and genetic algorithms (GA) not only predict environmental fate and toxicity but also simulate simultaneous biodegradation processes. When conventional bioremediation fails to sufficiently reduce the impact of persistent, resistant pollutants, in‐silico remediation techniques offer a promising alternative. By utilising well-defined model systems and algorithm-based programmes, such challenges can be effectively addressed (Singh et al., 2021).
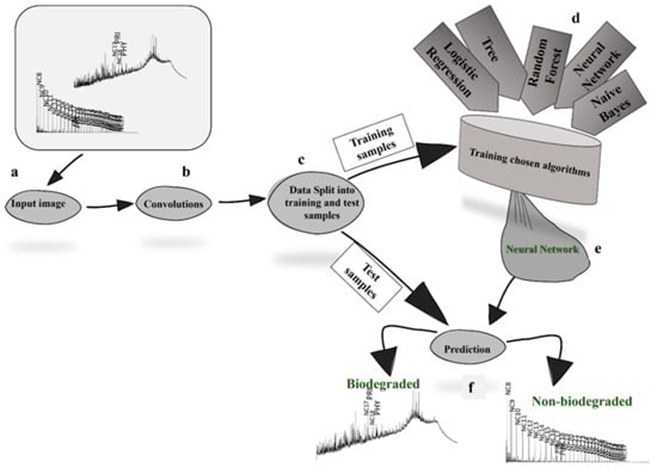
Figure 4: Orange® software’s image analysis feature: (a) input image; (b) convolutional computations; (c) separation of test and training samples; (d) training of five algorithms; (e) testing of the optimal model; (f) output classification (Bispo-Silva et al., 2023).
Artificial intelligence (AI) endows machines with the ability to operate intelligently and make decisions based on inputs, without the need for explicit external instructions typically supplied to conventional computers for specific tasks. AI systems are built upon models that are often pre-trained prior to making predictions. Common AI tools include boosted regression trees (BRT), immune algorithms (IA), ant colony algorithms (ACA), artificial neural networks (ANN) and Monte Carlo simulations (MCS). These tools are increasingly utilised to monitor environmental quality, particularly by facilitating the interpretation of high-dimensional and nonlinear data through advanced machine learning techniques.
Several studies have demonstrated the optimisation of factors crucial to the microbial biodegradation of petroleum hydrocarbons using standard mathematical and statistical models. For instance, Bordoloi et al. (2014) employed response surface modelling (RSM) to optimise growth conditions for the desulphurisation of dibenzothiophene (DBT)—a compound predominantly found in diesel oil—by Achromobacter sp. isolated from petroleum-contaminated soil. Similarly, Ramasamy et al. (2017) utilised RSM to optimise conditions for culturing Enterobacter cloacae (KU923381) to degrade diesel oil. Despite the extensive use of statistical models and traditional mathematical algorithms in studies of hydrocarbon degradation, only a few investigations have integrated AI for hydrocarbon cleanup and monitoring. Figure 5 illustrates the increasing role of AI in the bioremediation of hydrocarbon-contaminated sites over the past ten years (Patowary et al., 2023).
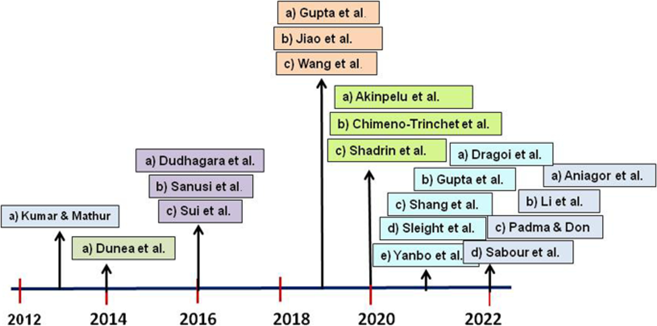
Figure 5: The use of AI in hydrocarbon cleanup over a ten-year period (2012–2022) (Patowary et al., 2023).
Kumar and Mathur (2013) opted for an ANN to model the in-situ biodegradation of hydrocarbons, rather than relying on the computationally intensive BIOPLUME III model. Although the two-dimensional finite difference model BIOPLUME III is capable of simulating both aerobic and anaerobic hydrocarbon biodegradation processes, its lengthy computation times necessitate the use of proxy models. In their study, the Levenberg–Marquardt back propagation technique was employed to develop an ANN that significantly improved efficiency.
Dunea et al. (2014) developed a decision support system—termed eSCAP (soil petroleum contamination assessment prototype)—to assist users in monitoring contaminant release during petroleum extraction and transportation. The system selects the most appropriate and practical remediation technologies from a comprehensive database, which is critical for successful remediation, as evidenced by its application in several oil spill case studies resulting from transportation leaks.
Dudhagara et al. (2016) further enhanced the degradation of fluoranthene by Mycobacterium litorale by employing two models: an improved ANN and a traditional RSM approach. Their study optimised media components—including NH₄NO₃, KH₂PO₄, and CaCl₂—by training multiple ANN topologies and selecting the best model based on minimisation of the root mean square error (RMSE) and mean absolute percentage error (MAPE). The ANN model effectively replicated the fluoranthene degradation process, yielding more accurate, consistent, and repeatable results due to its capacity to model nonlinear relationships, in contrast to RSM models that rely solely on quadratic equations. Notably, the ANN model achieved a degradation of 51.28% on the third day, compared with only 26.37% degradation after seven days using an unoptimised approach (Patowary et al., 2023).
In another study, Sanusi et al. (2016) conducted a comparative analysis of RSM and ANN to optimise the breakdown of total petroleum hydrocarbons (TPH) by the tropical plant Paspalum scrobiculatum in diesel-contaminated soil. Under RSM, optimum conditions were predicted at a diesel concentration of 3%, 72 sampling days, and an aeration rate of 1.77 mL/min, resulting in 76.8% TPH removal. In contrast, the ANN predicted optimum conditions at the same diesel concentration and sampling days but with an aeration rate of 1.02 mL/min, leading to 85.5% TPH removal. These findings confirm that the ANN outperforms the traditional RSM model in both prediction accuracy and data fitting.
AI also serves as an effective tool for tracking the spatial distribution of environmental contaminants. For example, Sui et al. (2016) examined the adsorption and diffusion of sixteen polyaromatic hydrocarbons (PAHs) in silica nanopores using molecular dynamics simulation, considering factors such as adsorption energy, free surface area, mean square displacement, and volume fraction. Their analysis indicated that diffusion is the primary mechanism driving PAH sorption in silica nanopores. To further quantify the impact of various parameters on adsorption, they employed the partial least squares (PLS) technique and multiple machine learning methods—including support vector regression (SVR), M5 decision trees (M5P), and multilayer perceptrons (MLP)—to conduct both linear and nonlinear regression. This combined approach of machine learning and molecular dynamics (MD) enhances our understanding of how organic pollutants are sequestered in soil particles.
To improve the precision of image analysis, an Otsu algorithm was applied to reduce noise in the observed images, and a maximum stable extremal region algorithm (MSERA) was employed to extract polygonal features from the detection boxes. Compared to conventional detection approaches, this method successfully identified oil spill regions while reducing the cost of detection by 57.2% (Patowary et al., 2023).
Wang et al. (2019) further demonstrated the utility of AI by employing an integrated extended short-term memory network (LSTM) to identify point sources of pollution and track industrial toxins in water bodies. Using cross-correlation and association rules (Apriori), they generated cross-correlation maps of water quality that aided in pinpointing contamination sources. In a separate study, Shadrin et al. (2020) predicted the phytotoxicity of TPH using various machine learning models—including support vector machines (SVM) and ANN—based on data from eleven soil samples collected from the Sakhalin Islands under greenhouse conditions. These models effectively forecasted the phytotoxic effects of TPH and facilitated the analysis of soil quality, a typically time-consuming and labour-intensive process.
Additionally, Dragoi et al. (2021) employed a neuro-evolutive methodology that combined ANN and differential evolution (DE) to forecast TPH and organic carbon (OC) levels, which are key indicators of the efficiency of oily sludge composting. The predictions from the ANN model were validated against experimental data, demonstrating consistency with observed values.
AI has also been applied to interpret the rheological properties that influence the transportability and refining of petroleum. An ANN-based model was recently utilised by Stratiev et al. (2023) to forecast the viscosity of crude oil. Collectively, these examples underscore the broad applicability of AI in identifying oil pollution sites and enhancing the accuracy of specific remediation procedures, thereby rendering them more efficient.
In summary, integrating AI with traditional remediation technologies improves the accuracy, speed, and cost-effectiveness of restoration processes. Recent trends indicate a significant increase in AI-based remedial research. Moreover, sophisticated bioinformatics methods now allow for detailed studies of hydrocarbon breakdown patterns and the catabolic pathways utilised by microbes in contaminated environments. In the future, it may be possible to develop a comprehensive database containing detailed data on various oil-contaminated sites, providing invaluable insights to environmental researchers and practitioners. Consequently, research on AI-based bioremediation is poised to play a pivotal role in addressing complex environmental challenges.
Bibliometric analysis—an approach that quantitatively assesses the influence and interrelationships among publications using statistical and mathematical methods (Lee et al., 2020)—offers an additional means of visualising academic output through publication and citation data. Such bibliometric mapping can reveal relationships, thereby generating new ideas and discoveries. Although research on oil bioremediation has not yet undergone extensive bibliometric mapping or clustering analysis, early studies in this field have focused on bacterial species that degrade hydrocarbons and the toxicity of hydrocarbons to Antarctic species. Over the 2000s and 2010s, studies on bioremediation employing various microorganisms, as well as in-depth molecular and physiological analyses of hydrocarbon-degrading species, gained prominence (Lo Giudice et al., 2010). Recent technological advancements—encompassing gene analysis, microcosm studies, microbial community research, and biomonitoring investigations—promise to further enhance bioremediation strategies (Rizzo et al., 2019).
4. Case Studies and Applications
Researchers and practitioners can enhance the microbial decomposition of oil contamination by utilising artificial intelligence (AI) to inform more effective bioremediation procedures and reduce environmental impact. Numerous studies have demonstrated that the microbiological degradation of oil pollutants can be successfully predicted and enhanced using AI. While traditional mathematical and statistical models that optimise various factors have significantly contributed to understanding the biodegradation of petroleum hydrocarbons, relatively few studies have employed AI for hydrocarbon cleanup and monitoring.
The following case studies illustrate how AI can be utilised to forecast and enhance the microbial degradation of oil contamination through computer models, laboratory-based research, and field applications.
4.1 Field Applications
AI has proven to be a valuable tool for tracking the spatial distribution of environmental contaminants. For example, Sui et al. (2016) employed molecular dynamics modelling to quantify parameters such as adsorption energy, free surface area, mean square displacement, and volume fraction in order to study the adsorption and diffusion of sixteen polyaromatic hydrocarbons (PAHs) in silica nanopores. Their analysis concluded that diffusion is the primary mechanism responsible for the sorption of PAHs in these nanopores. To quantify the influence of various parameters on adsorption, they applied both linear and nonlinear regression using the partial least squares (PLS) approach in conjunction with machine learning algorithms. Their results suggest that combining machine learning with molecular dynamics (MD) can significantly enhance our understanding of how organic pollutants are sequestered in soil particles.
In another study, Jiao et al. (2019) developed an innovative technique for the automatic detection of oil spills, which are typically challenging to identify. Their approach consisted of three components: a deep convolutional neural network (CNN) for image-based identification, conventional algorithms to eliminate noise (using an Otsu algorithm), and unmanned aerial vehicles (UAVs) for real-time monitoring. To extract precise geometric features, a maximum stable extremal region algorithm (MSERA) was applied to delineate the spill boundaries. Their method successfully identified oil spill regions and reduced detection costs by 57.2% compared with traditional detection methods.
Wang et al. (2019) further demonstrated the field application of AI by employing an integrated extended short-term memory network (LSTM) to identify point sources of pollution and track industrial toxins in water bodies. Using cross-correlation and association rules (Apriori), they created cross-correlation maps of water quality that assisted in tracking the origins of contamination.
4.2 Laboratory-Based Research
In addition to field applications, AI has been effectively employed in laboratory-based research to predict and enhance the microbial degradation of oil pollutants. Various experimental designs—including batch experiments, continuous cultures, microcosm studies, mesocosm studies, and omics-based analyses (e.g., genomics, transcriptomics, and metabolomics)—have been used to assess microbial activity.
For instance, Sanusi et al. (2016) conducted a comparative study using response surface methodology (RSM) and artificial neural networks (ANN) to evaluate the efficacy of Paspalum scrobiculatum, a tropical plant, for pilot-scale phytoremediation of diesel-contaminated sand. Using a central composite design, they optimised variables such as diesel concentration, sampling duration, and aeration rate. The ANN predicted optimum conditions at a diesel concentration of 3%, 72 sampling days, and 1.02 mL/min aeration, resulting in 85.5% removal of total petroleum hydrocarbons (TPH). In contrast, the RSM approach predicted optimum conditions at the same diesel concentration and sampling days but with an aeration rate of 1.77 mL/min, achieving 76.8% TPH removal. These findings confirm that the ANN model outperforms the traditional RSM model in terms of estimation accuracy and data fitting.
Similarly, Ramasamy et al. (2017) employed RSM to optimise several growth conditions for cultures of Enterobacter cloacae (KU923381) aimed at degrading diesel oil.
Real-world simulation models also serve as important case studies. Instead of using the computationally intensive BIOPLUME III, Kumar and Mathur (2013) employed an ANN to model the in situ biodegradation of hydrocarbons. By utilising the Levenberg–Marquardt back propagation technique, they developed a proxy model that provided improved efficiency in simulating both aerobic and anaerobic biodegradation processes.
Dunea et al. (2014) developed a decision support system, known as eSCAP (soil petroleum contamination assessment prototype), which monitors contaminant release during petroleum extraction and transportation. The system then selects the most appropriate remediation technology from a comprehensive database. This approach has been successfully applied in several case studies of oil spills resulting from transportation leaks.
Furthermore, Dudhagara et al. (2016) investigated the degradation of fluoranthene by Mycobacterium litorale using two models: an enhanced ANN and traditional RSM. They optimised media components—including NH₄NO₃, KH₂PO₄, and CaCl₂—by training multiple ANN topologies and selecting the best model based on minimisation of the root mean square error (RMSE) and mean absolute percentage error (MAPE). Their results demonstrated that the ANN model replicated the fluoranthene degradation process with greater accuracy, consistency, and repeatability than RSM, achieving 51.28% degradation on the third day compared with only 26.37% after seven days using an unoptimised approach.
5. Challenges and Future Directions
AI is increasingly used in predictive microbial degradation of oil contamination to enhance the efficacy and efficiency of bioremediation processes. By integrating the natural metabolic activities of bacteria with the predictive power of AI, researchers can better control and anticipate the outcomes of biodegradation. However, several challenges remain.
5.1 Data Scarcity and Quality
One major challenge is the scarcity of large, diverse, high-quality datasets necessary for training robust AI models. Many datasets in microbial bioremediation are sparse, fragmented, or inadequately standardised. For example, while genomic sequencing of oil-degrading microbes such as Alcanivorax borkumensis and Pseudomonas has advanced, there is limited data regarding their interactions with other species and environmental factors under different oil contamination scenarios (Yakimov et al., 2007).
5.2 Biological Complexity
The intricate interactions within microbial ecosystems represent a significant challenge for AI-driven predictions. Microbial degradation involves both cooperative and competitive interactions among species, which are highly context-dependent and influenced by the chemical composition of the oil spill and prevailing environmental conditions. Current machine learning algorithms often struggle to capture these non-linear and dynamic interactions (Varjani & Upasani, 2016).
5.3 Interdisciplinary Collaboration
Effective integration of microbiological, environmental, and computational data is essential but can be hindered by differences in methodologies and terminologies across disciplines. Such interdisciplinary barriers can slow collaborative efforts and the development of comprehensive models (Steinberger et al., 2021; Adams et al., 2022).
5.4 Model Explainability and Generalisation
Many advanced AI algorithms, particularly in deep learning, function as “black boxes”, which makes it difficult to interpret how predictions are generated. This lack of transparency can lead to mistrust among environmental stakeholders and regulatory bodies, who require clear explanations for decision-making (Adadi & Berrada, 2018). Furthermore, AI models trained on data from a specific oil spill scenario may not generalise well to other contexts, limiting their broader applicability.
5.5 Data Governance and Ethical Considerations
Effective data governance is crucial to avoid issues related to data security, integrity, and privacy. Inadequate data management can lead to breaches or loss of valuable data, particularly in projects involving sensitive or proprietary information. Ethical concerns, including data ownership, the environmental cost of AI deployment, and potential job displacement, also need to be addressed (Vinuesa et al., 2020).
Future research should prioritise the creation of standardised, extensive datasets specifically for oil-degrading microbial strains, develop AI models capable of capturing the complex dynamics of microbial communities, and foster interdisciplinary collaboration. Additionally, establishing comprehensive ethical guidelines and engaging with policymakers will be essential to facilitate responsible AI applications in environmental bioremediation.
6. Conclusion
The transformative potential of AI in everyday life—from surgical operations in medicine to environmental bioremediation—is now evident. The application of AI in enhancing the microbial degradation of petroleum contamination is a critical development in environmental management. By integrating AI-driven predictive models with traditional bioremediation techniques, we can achieve more accurate, efficient, and cost-effective restoration processes. There is an urgent need for continued research, robust interdisciplinary collaboration, and the integration of AI into bioremediation practices to address the complex challenges posed by oil contamination. Embracing these advances will not only improve remediation outcomes but also contribute to the development of sustainable strategies for protecting and restoring our environment.
Conflict of Interest
The author declares no conflict of interest.
References
Adadi, A., & Berrada, M. (2018). Peeking inside the black-box: A survey on explainable artificial intelligence (XAI). IEEE Access, 6, 52138–52160. https://doi.org/10.1109/ACCESS.2018.2870052
Adams, J., Carter, S., & Nguyen, H. (2022). Overcoming communication barriers in interdisciplinary research: A case study. Science and Public Policy, 49(4), 567–579. https://doi.org/10.1093/scipol/scac015
Adetitun, D. O. (2020). Different approaches to the biodegradation of hydrocarbons in halophilic and non-halophilic environments. In Current Microbiological Research in Africa: Selected Applications for Sustainable Environmental Management (pp. 313–331).
Amini, F., Giyahchi, M., & Moghimi, H. (2024). Bioremediation of petroleum contamination by microorganisms: Role of microbial communities and applications. In Microbial Bioremediation and Multiomics Technologies for Sustainable Development: Recent Trends (p. 136).
Barbinta-Patrascu, M. E., Bita, B., & Negut, I. (2024). From nature to technology: Exploring the potential of plant-based materials and modified plants in biomimetics, bionics, and green innovations. Biomimetics, 9(7), 390.
Baskaran, D., & Byun, H. S. (2024). Current trend of polycyclic aromatic hydrocarbon bioremediation: Mechanism, artificial mixed microbial strategy, machine learning, ground application, cost and policy implications. Chemical Engineering Journal, 155334.
Baum, Z. J., Yu, X., Ayala, P. Y., et al. (2021). Artificial intelligence in chemistry: Current trends and future directions. Journal of Chemical Information and Modelling, 61, 3197–3212.
Bispo-Silva, S., Ferreira de Oliveira, C. J., & Barberes, G. (2023). Geochemical biodegraded oil classification using a machine learning approach. GeoSciences, 13(11), 321.
Bordoloi, N. K., Rai, S. K., Chaudhuri, M. K., & Mukherjee, A. K. (2014). Deep-desulphurisation of dibenzothiophene and its derivatives present in diesel oil by a newly isolated bacterium Achromobacter sp. to reduce the environmental pollution from fossil fuel combustion. Fuel Processing Technology, 119, 236–244.
Chung, T. H., & Dhar, B. R. (2024). Bioelectrochemical sensors for detecting recalcitrant and toxic organic pollutants. In Emerging Trends and Advances in Microbial Electrochemical Technologies (pp. 201–221). Elsevier.
Dragoi, E. N., Godini, K., & Koolivand, A. (2021). Modelling of oily sludge composting process by using artificial neural networks and differential evolution: Prediction of removal of petroleum hydrocarbons and organic carbon. Environmental Technology & Innovation, 21, 101338.
Dudhagara, D. R., Rajpara, R. K., Bhatt, J. K., Gosai, H. B., & Dave, B. P. (2016). Bioengineering for polycyclic aromatic hydrocarbon degradation by Mycobacterium litorale: Statistical and artificial neural network (ANN) approach. Chemometrics and Intelligent Laboratory Systems, 159, 155–163.
Dunea, D., Iordache, S., & Pohoata, A. (2014). Investigation and selection of remediation technologies for petroleum-contaminated soils using a decision support system. Water, Air, & Soil Pollution, 7, 1–8.
Fan, M., Hu, J., & Cao, R. (2018). A review on experimental design for pollutants removal in water treatment with the aid of artificial intelligence. Chemosphere, 200, 330–343.
Gertsen, M. M., Arlyapov, V. A., Perelomov, L. V., Kharkova, A. S., Golysheva, A. N., Atroshchenko, Y. M., et al. (2024). Environmental implications of energy sources: A review on technologies for cleaning oil-contaminated ecosystems. Energies, 17(14).
Hemmati, A., Amiri, F., & Kouhgardi, E. (2024). Environmental sensitivity index mapping for the oil spill at a heavily industrialised area on the northern coast of the Persian Gulf. Journal of Coastal Conservation, 28(1), 17.
Jiao, Z., Jia, G., & Cai, Y. (2019). A new approach to oil spill detection that combines deep learning with unmanned aerial vehicles. Computers & Industrial Engineering, 135, 1300–1311.
Kuhn, M., Weston, S., Keefer, C., & Coulter, N. (2012). Cubist models for regression [R package vignette]. R package version 0.0, 18.
Kumar, D., & Mathur, S. (2013). Proxy simulation of in-situ bioremediation system using artificial neural network. International Journal of Computer Applications, 975, 8887.
Kuppan, N., Padman, M., Mahadeva, M., Srinivasan, S., & Devarajan, R. (2024). A comprehensive review of sustainable bioremediation techniques: Eco friendly solutions for waste and pollution management. Waste Management Bulletin.
Lee, I. S., Lee, H., Chen, Y. H., & Chae, Y. (2020). Bibliometric analysis of research assessing the use of acupuncture for pain treatment over the past 20 years. Journal of Pain Research, 13, 367–376.
Li, H., Zhou, Z., Long, T., Wei, T., Xu, J., Liu, S., & Wang, X. (2022). Big-data analysis and machine learning based on oil pollution remediation cases from CERCLA database. Energies, 15(15), 5698.
Liu, L., Liu, C., Fu, R., Nie, F., Zuo, W., Tian, Y., & Zhang, J. (2024). Full-chain analysis on emerging contaminants in soil: Source, migration and remediation. Chemosphere, 142854.
Lo Giudice, A., Casella, P., Caruso, C., Mangano, S., Bruni, V., De Domenico, M., & Michaud, L. (2010). Occurrence and characterization of psychrotolerant hydrocarbon-oxidizing bacteria from surface seawater along the Victoria Land coast (Antarctica). Polar Biology, 33, 929–943.
Lyu, X., Nuhu, M., Candry, P., Wolfanger, J., Betenbaugh, M., Saldivar, A., et al. (2024). Top-down and bottom-up microbiome engineering approaches to enable biomanufacturing from waste biomass. Journal of Industrial Microbiology and Biotechnology, kuae025.
Navina, B. K., Velmurugan, N. K., Kumar, P. S., Rangasamy, G., Palanivelu, J., Thamarai, P., et al. (2024). Fungal bioremediation approaches for the removal of toxic pollutants: Mechanistic understanding for biorefinery applications. Chemosphere, 350, 141123.
Paramesha, M., Rane, N. L., & Rane, J. (2024). Big data analytics, artificial intelligence, machine learning, internet of things, and blockchain for enhanced business intelligence. Partners Universal Multidisciplinary Research Journal, 1(2), 110–133.
Patowary, R., Devi, A., & Mukherjee, A. K. (2023). Advanced bioremediation by an amalgamation of nanotechnology and modern artificial intelligence for efficient restoration of crude petroleum oil-contaminated sites: A prospective study. Environmental Science and Pollution Research, 30, 74459–74484.
Ramasamy, S., Arumugam, A., & Chandran, P. (2017). Optimisation of Enterobacter cloacae (KU923381) for diesel oil degradation using response surface methodology (RSM). Journal of Microbiology, 55, 104–111.
Rawal, R. S., Naseem, S., Pandey, D., & Suman, S. K. (2024). Microbe-assisted remediation of xenobiotics: A sustainable solution. In Microbiome-Based Decontamination of Environmental Pollutants (pp. 317–355). Academic Press.
Rizzo, C. C., Malavenda, R., Gerçe, B., Papale, M., Syldatk, C., Hausmann, R., Bruni, V., Michaud, L., & Guidice, A. L. (2019). Effects of a simulated acute oil spillage on bacterial communities from Arctic and Antarctic marine sediments. Microorganisms, 7(12), 632.
Sanusi, S. N. A., Halmi, M. I. E., Abdullah, S. R. S., Hassan, H. A., Hamzar, F. M., & Idris, M. (2016). Comparative process optimisation of pilot-scale total petroleum hydrocarbon (TPH) degradation by Paspalum scrobiculatum L. Hack using response surface methodology (RSM) and artificial neural networks (ANNs). Ecological Engineering, 97, 524–534.
Shadrin, D., Pukalchik, M., & Kovaleva, E. (2020). Artificial intelligence models to predict acute phytotoxicity in petroleum contaminated soils. Ecotoxicology and Environmental Safety, 194, 110410.
Sharifani, K., & Amini, A. (2023). Machine learning and deep learning: A review of methods and applications. World Information Technology and Engineering Journal, 10(7), 3897–3904.
Siddique, A., Al Disi, Z., AlGhouti, M., & Zouari, N. (2024). Diversity of hydrocarbon-degrading bacteria in mangrove rhizosphere as an indicator of oil-pollution bioremediation in mangrove forests. Marine Pollution Bulletin, 205, 116620.
Singh, A. K., Bilal, M., Iqbal, H. M. N., & Raj, A. (2021). Trends in predictive biodegradation for sustainable mitigation of environmental pollutants: Recent progress and future outlook. Science of the Total Environment, 770, 144561.
Singh, H., Bhardwaj, N., Arya, S. K., & Khatri, M. (2020). Environmental impacts of oil spills and their remediation by magnetic nanomaterials. Environmental Nanotechnology, Monitoring & Management, 14, 100305.
Steinberger, E., Roberts, A., & Zhao, L. (2021). Bridging disciplines for effective environmental monitoring: Challenges and opportunities. Environmental Research Letters, 16(8), 084012. https://doi.org/10.1088/1748-9326/ac1e7b
Taiwo, O. R., Onyeaka, H., Oladipo, E. K., Oloke, J. K., & Chukwugozie, D. C. (2024). Advancements in predictive microbiology: Integrating new technologies for efficient food safety models. International Journal of Microbiology, 2024(1), 6612162.
Tedesco, P., Balzano, S., Coppola, D., Esposito, F. P., de Pascale, D., & Denato, R. (2024). Bioremediation for the recovery of oil-polluted marine environments: Opportunities and challenges approaching the blue growth. Marine Pollution Bulletin, 200, 116157.
Varjani, S. J., & Upasani, V. N. (2016). Biodegradation of petroleum hydrocarbons by an oleophilic strain of Pseudomonas aeruginosa (NCIM 5514). Bioresource Technology, 222, 195–201.
Vinuesa, R., Azizpour, H., Leite, I. M., Virginia, D. M., Sami, D. H., Anna, F., Simone, D. S., Max, T. K., & Francesco, F. N. (2020). The role of artificial intelligence in achieving the Sustainable Development Goals. Nature Communications, 11, 233. https://doi.org/10.1038/s41467-019-14108-y
Wang, P., Yao, J., Wang, G., Hao, F., Shresthra, S., Xue, B., Xie, G., & Peny, Y. (2019). Exploring the application of artificial intelligence technology for identification of water pollution characteristics and tracing the source of water quality pollutants. Science of the Total Environment, 693, 133440.
Yakimov, M. M., Timmis, K. N., & Golyshin, P. N. (2007). Obligate oil-degrading marine bacteria. Current Opinion in Biotechnology, 18(3), 257–266. https://doi.org/10.1016/j.copbio.2007.04.006
About this Article
Cite this Article
APA
Akinsemolu A.A. (2025).Artificial Intelligence (AI) in Predictive Microbial Degradation of Oil Contamination. In Akinyele B.J., Kayode R. & Akinsemolu A.A. (Eds.), Microbes, Mentorship, and Beyond: A Festschrift in Honour of Professor F.A. Akinyosoye. SustainE
Chicago
Akinsemolu A.A. 2025. “Artificial Intelligence (AI) in Predictive Microbial Degradation of Oil Contamination” In Microbes, Mentorship, and Beyond: A Festschrift in Honour of Professor F.A. Akinyosoye, edited by Akinyele B.J., Kayode R. and Akinsemolu A.A., SustainE.
Received
20 December 2024
Accepted
30 January 2025
Published
4 February 2025
Corresponding Author Email: adetitun.do@unilorin.edu.ng; adetitun.do@gmail.com
Disclaimer: The opinions and statements expressed in this article are the authors’ sole responsibility and do not necessarily reflect the viewpoints of their affiliated organizations, the publisher, the hosted journal, the editors, or the reviewers. Furthermore, any product evaluated in this article or claims made by its manufacturer are not guaranteed or endorsed by the publisher.
Distributed under Creative Commons CC-BY 4.0
Share this article
Use the buttons below to share the article on desired platforms.